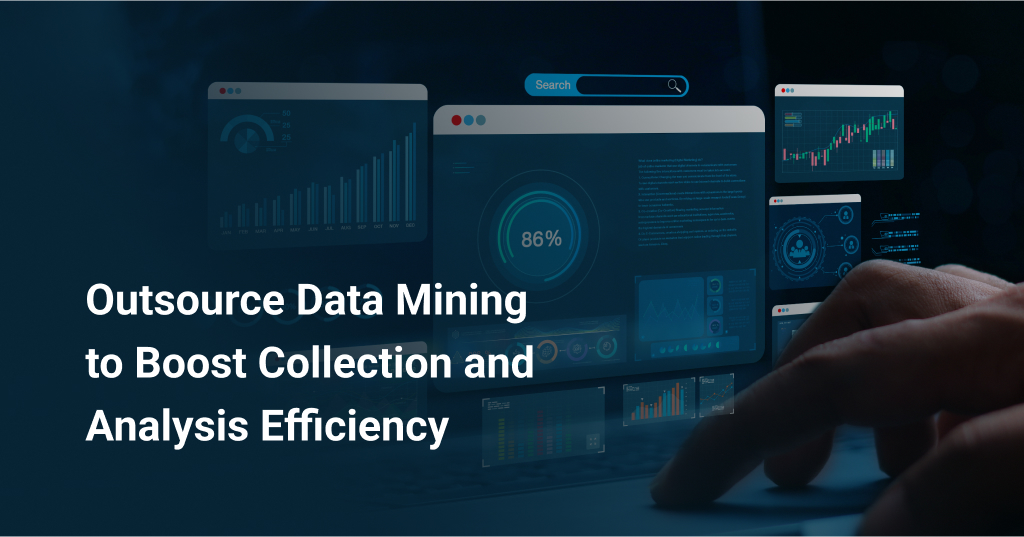
To become data-driven, companies often face difficulties in collecting and analyzing their data. However, the real challenge lies in integrating diverse data sources into a unified, actionable framework.
Data collection has become complex due to fragmented sources and incompatible formats. Furthermore, many high-value data sources are protected by anti-scraping measures, complicating access. As a result, teams spend too much time gathering and preparing data, delaying analysis and decision-making. The problem worsens when the internal team lacks the necessary tools or time to manage large-scale data operations, resulting in recurring inefficiencies and workflow disruptions.
Outsourcing data mining services can help streamline operations and ensure that the data is ready for strategic analysis. Here’s how this approach is helping businesses collect and leverage their data more effectively.
Why In-House Data Mining Fails to Scale: Common Challenges Faced by Teams
1. Difficulty Accessing Diverse Data Sources
Companies struggle to access reliable external data sources that often use anti-scraping measures or require expensive API subscriptions. In-house teams often lack the specialized tools or knowledge to handle CAPTCHAs, IP blocking, and other anti-scraping measures effectively. Without comprehensive data collection capabilities, teams struggle to support their strategic planning and operational decision-making.
2. Inability to Handle Multi-format Unstructured Data
Business data comes from various sources (like PDFs, dynamic web pages, scanned documents, or proprietary databases) in countless formats- CSV, JSON, XML, and unstructured text. Internal teams often lack tools or frameworks to extract, structure, and normalize this kind of data efficiently. Moreover, building parsers that can adapt to varied formats requires advanced tools and a structured approach—something most in-house teams don’t have the time to build. Without adaptable tools and scalable frameworks, in-house setups often struggle to keep pace—resulting in significant delays between data collection and insight generation.
3. High Cost of Infrastructure Maintenance
Even when in-house teams build functional data mining pipelines, maintaining them becomes a full-time job. APIs change, websites implement new bot protections, and data formats evolve. Keeping scripts up to date, re-training parsers, or fixing failures diverts technical resources from innovation to maintenance. This ongoing maintenance is costly and time-consuming, delaying data processing and analysis.
4. Compliance, Ethics, and Legal Risk
Complying with data privacy regulations (like GDPR, CCPA) or a platform’s terms of service is complex due to varying restrictions, legal requirements, and enforcement policies across different providers. Internal teams may scrape or extract data without understanding the legal implications, putting the business at risk. Without vetting processes or data compliance frameworks, in-house efforts could lead to privacy regulations violations, blacklisting, or even legal action—risks that many teams underestimate.
5. In-House Systems Fall Short as Data Volumes Grow
As businesses grow, their in-house data collection methods often fail to keep pace. Systems built for smaller datasets often lack the scalability to manage growing data volumes efficiently. The infrastructure upgrades needed for this scale are typically reactive rather than proactive, creating persistent lag in data availability.
6. Lack of Data Governance Framework
Most in-house teams lack clear rules about who owns data, how it should be collected, and who can access it. In the absence of a governance framework, departments collect similar data inconsistently, complicating analysis. When there’s no defined process for data ownership, quality checks, or documentation, the risk of errors increases, and teams spend more time fixing issues than analyzing data.
How Outsourcing Improves Data Collection and Analysis Efficiency
Given the limitations of scaling data mining in-house, outsourcing data mining services has become a strategic move for businesses aiming to improve how they collect, process, and analyze data. Let’s explore how it offers a way to optimize data workflows, reallocate internal resources, and scale data operations without investing in full in-house capabilities:
1. Access to On-Demand Expertise Without Hiring Overhead
Experienced data mining service providers have dedicated teams of professionals who specialize in collecting critical information from complex and protected sources. Using custom scripts, APIs, and advanced tools, these teams manage the entire process — from data collection to enrichment and validation — providing structured, validated data efficiently and eliminating the need for extensive internal hiring or workforce training.
2. Scalable Infrastructure That Adapts to Business Needs
Data mining outsourcing companies have high-power computing systems or cloud-based resources built for handling large-scale data collection and processing. This eliminates the need for companies to constantly upgrade internal systems as their data grows and ensures reliable performance.
3. Quality Control Processes
Instead of checking data quality at the end of the collection process, data mining service providers build quality checks at every stage. They implement automated validation that immediately identifies outliers, inconsistencies, or changes in data formats, preventing errors from propagating into later stages. Additionally, their teams cross-validate data across multiple sources to ensure accuracy and completeness. This hybrid approach ensures that the data delivered is reliable and ready for analysis.
4. Multi-format Data Handling
Data mining solution providers use advanced parsing tools and flexible frameworks specifically designed to process data from various sources and formats—including structured (CSV, XML, JSON) and unstructured data (PDFs, images, or webpages). These systems efficiently standardize and integrate data into a consistent format, allowing companies to perform analysis quickly across most common data sources and formats.
5. Ensured Compliance from Day One
Data mining service providers follow strict protocols to comply with data privacy regulations (such as GDPR, CCPA) and each website’s terms of service. They implement legal frameworks that review and validate the terms of each website before initiating data scraping. This ensures that only publically available information is collected, following each site’s robots.txt rules and staying compliant with data protection laws. By maintaining clear documentation and compliance checks, they minimize legal risks while ensuring responsible data collection.
6. Built-In Maintenance and Support for Data Pipelines
Data mining solution providers handle the maintenance of data pipelines as part of their data collection services, ensuring that the data you receive is clean, accurate, and standardized for analysis. They proactively address issues and adjust to changes in data sources or website structures, freeing internal teams to focus on strategic initiatives like analysis, modeling, or forecasting. This includes updating API connections, reconfiguring scraping scripts to accommodate website updates, and ensuring consistent and reliable data collection.
Real-Life Examples of Successful Data Mining Outsourcing
Case Study 1: Reducing Data Collection Costs for an Energy Consulting Firm
Challenges Faced by Client:
The client struggled with collecting comprehensive retail energy pricing data across various providers due to diverse website structures and anti-scraping measures like CAPTCHAs and IP blocking.
Project Requirements:
They sought assistance in manually extracting detailed pricing information, including rates and terms for natural gas and electricity plans, ensuring data accuracy and consistency across multiple sources.
Project Outcomes:
SunTec Data deployed a dedicated team to collect the required data manually (using custom scripts and APIs) while bypassing anti-scraping barriers. The team also performed manual checks to enrich incomplete data. By filling the gaps in automated data extraction, we reduced the client’s overhead costs by 40%.
Case Study 2: Enhancing Medical Data Accuracy for a Healthcare Consulting Firm
Challenges Faced By Client:
The client was struggling to collect data on physicians, including practice locations and contact details, from various sources. Due to issues like incomplete or missing information, he felt a need for manual data extraction.
Project Requirements:
They required a customized list of U.S.-based physicians, necessitating data mining and enrichment services to extract and validate relevant information from multiple sources while ensuring HIPAA compliance.
Project Outcomes:
By supporting manual data extraction and validation, we helped the client acquire data 5X faster and improve accuracy by 35%.
Ready to Optimize Your Data Mining Strategy? Partner With Us
At SunTec Data, we understand that accurate, reliable data is the key to effective analysis and informed decision-making. Hence, our data mining services are built to address complex data extraction and compliance requirements. Using over two decades of industry experience, we’ve built the tools, processes, and expertise you need, eliminating the need for costly internal infrastructure or specialized hires. Contact us today to improve data efficiency, ensure compliance, and unlock actionable insights.